TEST ASSIMILATIONS OF THE REAL-TIME, MULTI-SENSOR
HOURLY PRECIPITATION ANALYSIS INTO THE NCEP ETA MODEL
1. Introduction
In 1996, NCEP developed an hourly, real-time 4km U.S.
precipitation analysis (Baldwin and Mitchell, 1997).
The analysis uses hourly precipitation
estimates from the WSR-88D radar network, and the hourly reports from
the approximately 2,500 automated rain gauges via the GOES Data Collection
Platform. This product gives us the opportunity to assimilate precipitation
observations in the mesoscale Eta model's 4-dimensional data assimilation
system (4DDA), in order to improve the quality of
- the 4DDA precipitation accuracy
- the short-term Eta model precipitation forecast
- the other water cycle components, such as soil moisture in the
4DDA and forecast product suite.
2. The Assimilation Method
The essence of precipitation assimilation in the Eta Data Assimilation
System (EDAS) is to use observed precipitation to adjust the model's latent
heating, moisture and cloud water fields during the 12-hour pre-forecast
assimilation period. To that end, at each time step during EDAS, for each
grid point where precipitation observations are available, we compare
the model precipitation (Pmod ) against the observations
(Pobs ) and make adjustments in the following way:
- If Pmod > 0 but Pobs = 0:
we take back the Pmod and the corresponding amount
of latent heating from the model; adjust water vapor mixing ratio
(qv) so the relative humidity (RH) remains
unchanged; and reduce cloud water mixing ratio to no more than the
minimum amount required to produce rain (qcmin).
- If Pmod > Pobs > 0: we reduce the
latent heat release in each precipitating layer by multiplying the
latent heating profile by the factor of
Pobs/Pmod; adjust
qv as in the previous scenario, and in layers
where precipitation is being produced, reduce cloud water
proportionally but keep it above the qcmin.
- Pmod < Pobs: first check to see
if convection is possible, if so, we shorten the convective time
scale to accelerate convective precipitation production,
with the goal of producing an amount (Pcnv)
that equals to Pobs (but no greater than the
maximum amount allowed by the convective parameterization).
After the convective adjustment, if
Pcnv < Pobs (either because
the profile is not convective, or the maximum possible convective
precipitation is less than Pobs), then we perform
a grid scale precipitation adjustment by comparing the model's
original grid scale precip (Pgrd )
against Pobs - Pcnv.
- If Pgrd > 0, we multiply the grid scale latent
heating profile by
Pobs-Pcnv/Pgrd,
change the qc in the rain-producing layers by the
same factor (but keep it above qcmin )
and adjust the qv so RH remains unchanged.
- If Pgrd = 0, we create a layer of cloud
(the upper and lower limits of the cloud layer is determined by the
model's moisture profile and the amount of target grid scale
precipitation) and a parabolic latent heating profile which
corresponds to the target grid-scale precipitation production
(Pobs-Pcnv ). Within the
cloud layer we created, RH is set to 80% and
qc is set to no less than qcmin.
3. The 1-15 Jul 1998 experiment
In the first half of July 1998, we ran a series of real-time experiments
to assimilate the multi-sensor hourly precipitation observations into
the 80-km version of the Eta model. The results are compared to a control
run, which is an 80-km Eta model that mimicks the 32-km operational Eta model
in everyway except the resolution. This series of experiments is later
re-run in retrospective mode to further refine the precipitation assimilation
method.
We find that precipitation assimilation has a significant positive
impact on the model's precipitation production during the 12h data assimilation
(EDAS) period, as shown below:
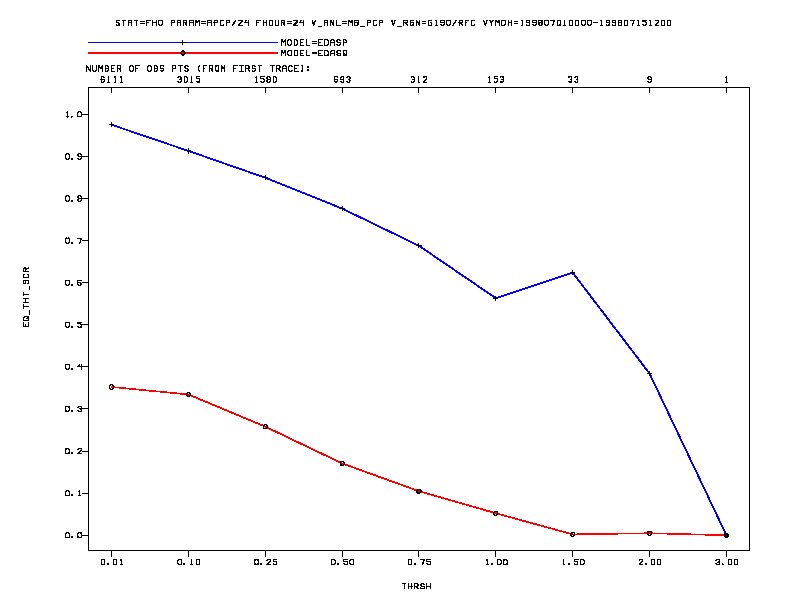
Fig. 1 Equitable threat scores (top) and bias scores (bottom)
for the precipitation experiment (blue) and the
control run (red) during the data assimilation period. The scores are
calculated by summing up the 00Z and 12Z cycles of 12h EDAS precipitation
(hence yielding a 12Z-12Z 24h sum) and comparing against the 24h sum of
multi-sensor hourly observations.
The total precipitation accumulation for the first half of Jul 1998 is shown
below, for the two model runs (control, and the precipitation assimilation),
and compared to the observed precipitation summed up from the River Forecast
Center 24-hour precipitation data:
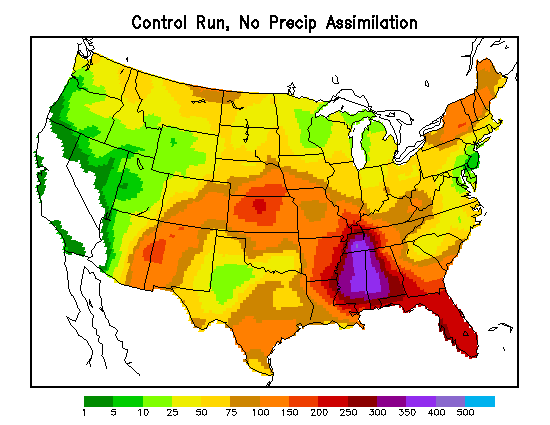
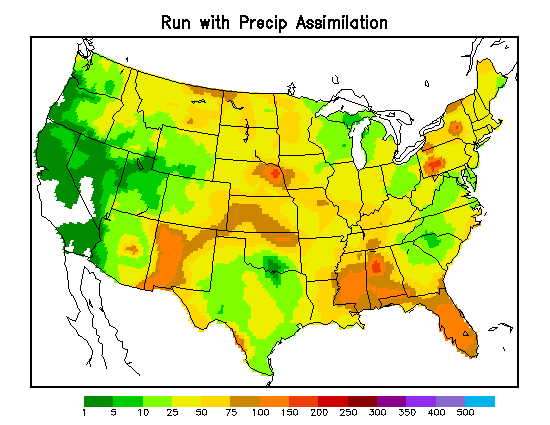
Fig. 2 Total precipitation accumulation for 12Z 30 Jun - 12Z 15 Jul
1998, from 1) The control run EDAS (top left), 2) the run with precipitation
assimilation (top right) and 3) 15-day sum of 24h precipitation observations
from the River Forecast Centers.
Compared to observations, the control run has a high precipitation bias in
the Southeast U.S., for the first half of Jul 1998. In contrast, the run with
precipitation assimilation produced an accumulated rainfall field that is much
closer to the observations. The difference in accumulated rainful over the
preceding 15 days leads to a large difference in soil moisture by 12Z 15 Jul
1998:
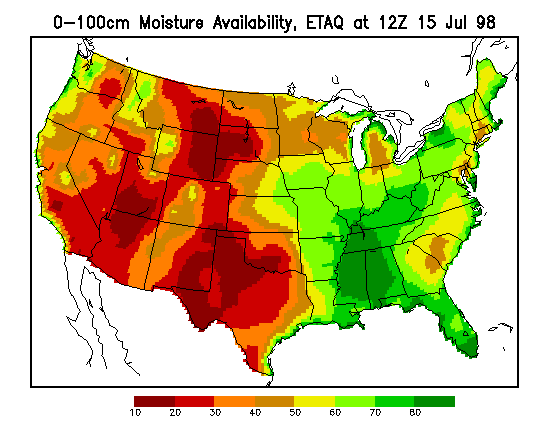
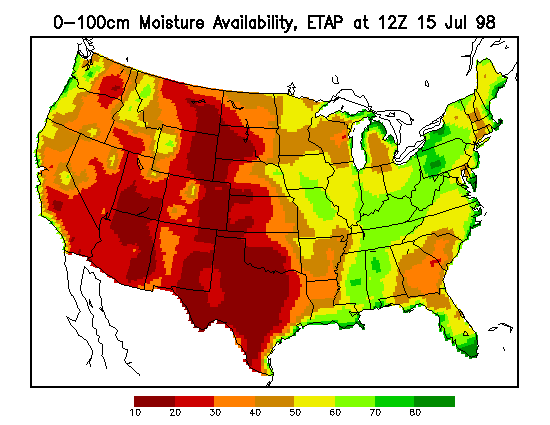
Fig. 3 0-100cm soil moisture availability at 12Z 15 Jul 1998, for
1) the control run (left panel), 2) the run with precipitation
assimilation (right panel).
The two series of runs started out with the identical soil moisture fields.
In the control run, the large area of wet soil in the southeast region
obviously resulted from the excessive rainfall in the area. The more-moderate
rainfall in the run with precipitation assimilation produced a more reasonable
soil moisture field after 15 days of model run.
We find that with the assimilation of hourly precipitation
data, the model's precipitation field during the 12h EDAS period closely
approximates the observations. The precipitation assimilation also improves
the model's rainfall field during the first several hours of free forecast.
It also has a very slight positive impact on longer-term forecasts, though
on occasion it produces a markedly better forecast, as shown in Fig. 4.
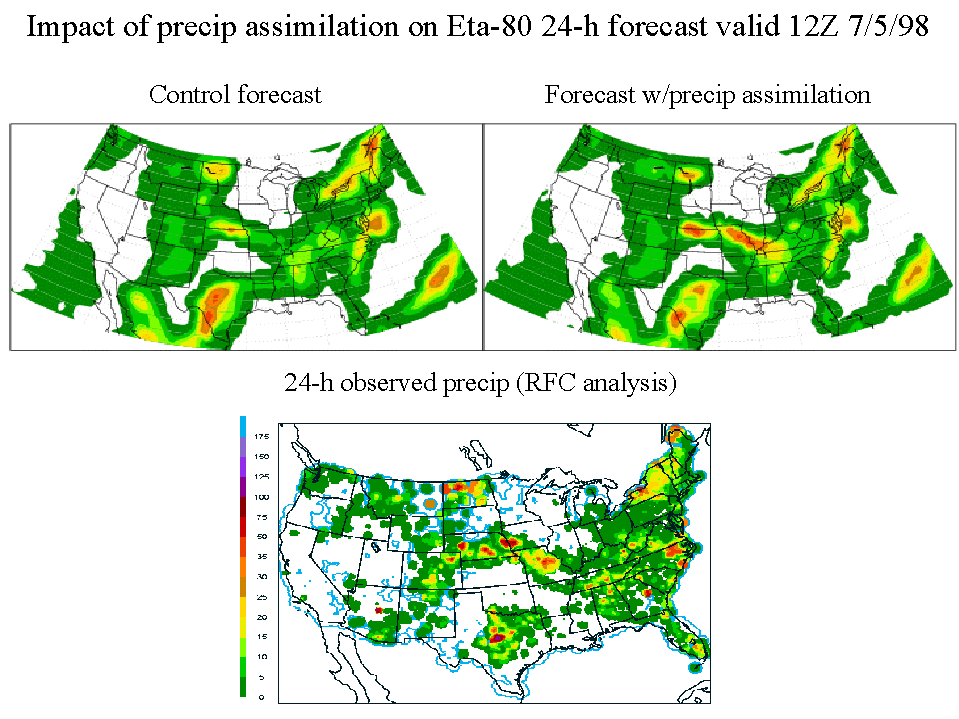
Fig. 4 24h precipitation accumulation ending 12Z 5 Jul 1998,
from the 24h forecast of the two model runs (top two figures), and from the
RFC 24h observations (bottom figure)
5. The 24 Jul 2001 Operational Implementation, and beyond
Precipitation assimilation was implemented into the operational Eta model
(22km/50 levels) on 24 Jul 2001 along with upgrades to land surface physics
and 3DVAR analysis.
On 27 Nov 2001, the Eta model underwent another upgrade,
where the grid resolution was increased to 12km/60 levels, a new cloud
microphysics package was implemented, and NOAA-16 radiances began to be
included in the 3DVAR analysis. The precipitation assimilation also
underwent extensive changes at this time to stay in tandem with the new
cloud microphysics.
24h Eta Forecast Threat and Bias scores for 2001, before and after
the 24 Jul implementation
24h Eta Forecast Threat and Bias scores for 25 Jul-31 Dec, 2000 vs. 2001
6. Discussion
We have found that precipitation assimilation produces a marked improvement
on model's atmospheric fields, in both the analysis and forecasts of 24
hours and beyond. It also improves the model's precipitation field
significantly during the 12h data assimilation period. This is quite useful
in providing an improved soil moisture field for the Eta model: currently
there is no real-time accessible nationwide network of soil moisture
observations available for assimilation, so an accurate precipitation
assimilation can help overcome this void.
The bias scores in Fig. 2 show that, during EDAS, while the precipitation
assimilation has corrected the control run's rather high bias in the larger
observed precipitation threshold values (0.5 to 2.0 in/day), it tends to
under-predict heavier rainfall by 40%-60%. This is largely due to the
low bias in the hourly data we use for the assimilation. We use the hourly
multi-sensor (largely radar-based) precipitation observations for our
assimilation, and evaluate the model's precipitation fields against the
24h RFC observations (largely gauge-based). In the past two years we have
routinely compared the multi-sensor hourly observations (summed over 24h
periods) against the 24h RFC observations, and found that the multi-sensor
observations tend to have false rainfall at very light amounts, while
under-estimating heavier rainfall by a percentage similar to that in the
EDAS bias scores (Fig. 2).
We plan to use the 24h RFC observations to correct this bias in the
hourly data, for the use in the precipitation assimilation. In retrospective
mode, this can be done by using the hourly data as ``weights'' to partition
the 24h observations and produce a more accurate set of hourly rainfall data.
In real time, this can be done by compiling a retrospective
database of the bias, in which we compare the two sets of observations
over the preceding
30-60 day period, and use this database to perform a bias correction
on the real-time hourly precipitation observations.
In the near future, we also plan to include GOES cloud heights observations
in the assimilation, to improve the model cloud fields, and to help reduce
the ``false light rain'' signal that radar data frequently bring to
the hourly precipitation observations.
ACKNOWLEDGMENT
Support for this research was provided by NOAA via the GEWEX Continental-Scale
International Project (GCIP), and by the University Corporation for
Atmospheric Research.
REFERENCES
Baldwin, M. E., and K.E. Mitchell, 1997: The NCEP hourly
multi-sensor U.S. precipitation analysis for operations and GCIP research.
Preprints, 13th AMS Conference on Hydrology, Long Beach, CA, 54--55.
Carr, F. H., and M. E. Baldwin, 1991: Assimilation of observed
precipitation data using NMC's Eta model. Preprints, 9th AMS Conference
on Numerical Weather Prediction, Denver, CO, 422-425.